初识MapReduce的应用场景(附JAVA和Python代码)
Java版本代码
先是准备一个数据集,包含着已经切割好的词汇,这里我们设置文件的格式是txt格式的。文件名是WordMRDemo.txt,内容是下面简短的一句话,以空格分割开:
hello my name is spacedong welcome to the spacedong thank you
引入Hadoop的依赖包
//这里使用的是2.6.5的依赖包,你可以使用其他版本的
<dependency>
<groupId>org.apache.hadoop</groupId>
<artifactId>hadoop-common</artifactId>
<version>2.6.5</version>
</dependency>
<dependency>
<groupId>org.apache.hadoop</groupId>
<artifactId>hadoop-client</artifactId>
<version>2.6.5</version>
</dependency>
(温馨提示:代码部分可左右滑动)
新建WordMapper.java文件,代码的作用是进行以空格的形式进行分词。
public class WordMapper extends Mapper<LongWritable, Text, Text, IntWritable> {
@Override
protected void map(LongWritable key, Text value, Mapper.Context context)
throws java.io.IOException, InterruptedException {
String line = value.toString();
//StringTokenizer默认按照空格来切
StringTokenizer st = new StringTokenizer(line);
while (st.hasMoreTokens()) {
String world = st.nextToken();
//map输出
context.write(new Text(world), new IntWritable(1));
}
}
}
新建WordReduce.java文件,作用是进行词汇的统计。
public class WordReduce extends Reducer<Text, IntWritable, Text, IntWritable> {
@Override
protected void reduce(Text key, Iterable<IntWritable> iterator, Context context)
throws java.io.IOException ,InterruptedException {
int sum = 0 ;
for(IntWritable i:iterator){
sum+=i.get();
}
context.write(key, new IntWritable(sum));
}
}
新建WordMRDemo.java文件,作用是运行Job,开始分析句子。
public class WordMRDemo {
public static void main(String[] args) {
Configuration conf = new Configuration();
//设置mapper的配置,既就是hadoop/conf/mapred-site.xml的配置信息
conf.set("mapred.job.tracker", "hadoop:9000");
try {
//新建一个Job工作
Job job = new Job(conf);
//设置运行类
job.setJarByClass(WordMRDemo.class);
//设置要执行的mapper类
job.setMapperClass(WordMapper.class);
//设置要执行的reduce类
job.setReducerClass(WordReduce.class);
//设置输出key的类型
job.setMapOutputKeyClass(Text.class);
//设置输出value的类型
job.setMapOutputValueClass(IntWritable.class);
//设置ruduce任务的个数,默认个数为一个(一般reduce的个数越多效率越高)
//job.setNumReduceTasks(2);
//mapreduce 输入数据的文件/目录,注意,这里可以输入的是目录。
FileInputFormat.addInputPath(job, new Path("F:BigDataWorkPlacedatainput"));
//mapreduce 执行后输出的数据目录,不能预先存在,否则会报错。
FileOutputFormat.setOutputPath(job, new Path("F:BigDataWorkPlacedataout"));
//执行完毕退出
System.exit(job.waitForCompletion(true) ? 0 : 1);
} catch (Exception e) {
e.printStackTrace();
}
}
}
最后执行WordMRDemo.java文件,然后得到的结果是out文件夹内的内容,它长这个样子:
out的文件目录
打开part-r-00000文件的内容如下
具体的文件内容Python代码版本
新建map.py文件,进行词汇的切割。
for line in sys.stdin:
time.sleep(1000)
ss = line.strip().split(' ')
for word in ss:
print ' '.join([word.strip(), '1'])
新建red.py文件,进行词汇的统计。
cur_word = None
sum = 0
for line in sys.stdin:
ss = line.strip().split(' ')
if len(ss) != 2:
continue
word, cnt = ss
if cur_word == None:
cur_word = word
if cur_word != word:
print ' '.join([cur_word, str(sum)])
cur_word = word
sum = 0
sum += int(cnt)
print ' '.join([cur_word, str(sum)])
新建run.sh文件,直接运行即可。
HADOOP_CMD="/usr/local/src/hadoop-2.6.5/bin/hadoop"
STREAM_JAR_PATH="/usr/local/src/hadoop-2.6.5/share/hadoop/tools/lib/hadoop-streaming-2.6.5.jar"
INPUT_FILE_PATH_1="/test.txt"
OUTPUT_PATH="/output"
$HADOOP_CMD fs -rmr -skipTrash $OUTPUT_PATH
# Step 1.
$HADOOP_CMD jar $STREAM_JAR_PATH
-input $INPUT_FILE_PATH_1
-output $OUTPUT_PATH
-mapper "python map.py"
-reducer "python red.py"
-file ./map.py
-file ./red.py
以上的是演示demo的核心代码,完整的代码可以上github的代码仓库上获取。
GitHub地址为:http://github.com/cassieeric/bigDaaNotes
以上的文章是MapReduce系列的第一篇,下篇预告是MapReduce的编程模型,敬请期待!
福利
看完后,是否对 MapReduce 有了初步的了解呢?最后送一本电子书给大家《Hadoop的技术内幕:深入解析MapReduce架构设计及实现原理》,在公众号后台回复 MapReduce 关键字即可获取。
参考资料:
Hadoop的技术内幕:深入解析MapReduce架构设计及实现原理
题图:cosmin Paduraru
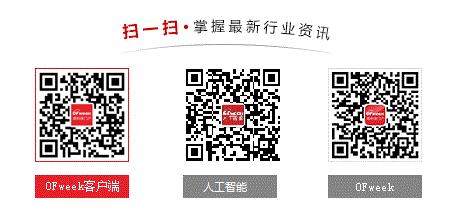
最新活动更多
-
即日-11.13立即报名>>> 【在线会议】多物理场仿真助跑新能源汽车
-
11月28日立即报名>>> 2024工程师系列—工业电子技术在线会议
-
12月19日立即报名>> 【线下会议】OFweek 2024(第九届)物联网产业大会
-
即日-12.26火热报名中>> OFweek2024中国智造CIO在线峰会
-
即日-2025.8.1立即下载>> 《2024智能制造产业高端化、智能化、绿色化发展蓝皮书》
-
精彩回顾立即查看>> 【限时免费下载】TE暖通空调系统高效可靠的组件解决方案
推荐专题
发表评论
请输入评论内容...
请输入评论/评论长度6~500个字
暂无评论
暂无评论