detectron2系列:config软件包
# ROI HEADS options
# ---------------------------------------------------------------------------- #
_C.MODEL.ROI_HEADS = CN()
_C.MODEL.ROI_HEADS.NAME = "Res5ROIHeads"
# Number of foreground classes
_C.MODEL.ROI_HEADS.NUM_CLASSES = 80
# Names of the input feature maps to be used by ROI heads
# Currently all heads (box, mask, ...) use the same input feature map list
# e.g., ["p2", "p3", "p4", "p5"] is commonly used for FPN
_C.MODEL.ROI_HEADS.IN_FEATURES = ["res4"]
# IOU overlap ratios [IOU_THRESHOLD]
# Overlap threshold for an RoI to be considered background (if < IOU_THRESHOLD)
# Overlap threshold for an RoI to be considered foreground (if >= IOU_THRESHOLD)
_C.MODEL.ROI_HEADS.IOU_THRESHOLDS = [0.5]
_C.MODEL.ROI_HEADS.IOU_LABELS = [0, 1]
# RoI minibatch size *per image* (number of regions of interest [ROIs])
# Total number of RoIs per training minibatch =
# ROI_HEADS.BATCH_SIZE_PER_IMAGE * SOLVER.IMS_PER_BATCH
# E.g., a common configuration is: 512 * 16 = 8192
_C.MODEL.ROI_HEADS.BATCH_SIZE_PER_IMAGE = 512
# Target fraction of RoI minibatch that is labeled foreground (i.e. class > 0)
_C.MODEL.ROI_HEADS.POSITIVE_FRACTION = 0.25
# Only used on test mode
# Minimum score threshold (assuming scores in a [0, 1] range); a value chosen to
# balance obtaining high recall with not having too many low precision
# detections that will slow down inference post processing steps (like NMS)
# A default threshold of 0.0 increases AP by ~0.2-0.3 but significantly slows down
# inference.
_C.MODEL.ROI_HEADS.SCORE_THRESH_TEST = 0.05
# Overlap threshold used for non-maximum suppression (suppress boxes with
# IoU >= this threshold)
_C.MODEL.ROI_HEADS.NMS_THRESH_TEST = 0.5
# If True, augment proposals with ground-truth boxes before sampling proposals to
# train ROI heads.
_C.MODEL.ROI_HEADS.PROPOSAL_APPEND_GT = True
# ---------------------------------------------------------------------------- #
# Box Head
# ---------------------------------------------------------------------------- #
_C.MODEL.ROI_BOX_HEAD = CN()
# C4 don't use head name option
# Options for non-C4 models: FastRCNNConvFCHead,
_C.MODEL.ROI_BOX_HEAD.NAME = ""
# Default weights on (dx, dy, dw, dh) for normalizing bbox regression targets
# These are empirically chosen to approximately lead to unit variance targets
_C.MODEL.ROI_BOX_HEAD.BBOX_REG_WEIGHTS = (10.0, 10.0, 5.0, 5.0)
# The transition point from L1 to L2 loss. Set to 0.0 to make the loss simply L1.
_C.MODEL.ROI_BOX_HEAD.SMOOTH_L1_BETA = 0.0
_C.MODEL.ROI_BOX_HEAD.POOLER_RESOLUTION = 14
_C.MODEL.ROI_BOX_HEAD.POOLER_SAMPLING_RATIO = 0
# Type of pooling operation applied to the incoming feature map for each RoI
_C.MODEL.ROI_BOX_HEAD.POOLER_TYPE = "ROIAlignV2"
_C.MODEL.ROI_BOX_HEAD.NUM_FC = 0
# Hidden layer dimension for FC layers in the RoI box head
_C.MODEL.ROI_BOX_HEAD.FC_DIM = 1024
_C.MODEL.ROI_BOX_HEAD.NUM_CONV = 0
# Channel dimension for Conv layers in the RoI box head
_C.MODEL.ROI_BOX_HEAD.CONV_DIM = 256
# Normalization method for the convolution layers.
# Options: "" (no norm), "GN", "SyncBN".
_C.MODEL.ROI_BOX_HEAD.NORM = ""
# Whether to use class agnostic for bbox regression
_C.MODEL.ROI_BOX_HEAD.CLS_AGNOSTIC_BBOX_REG = False
# If true, RoI heads use bounding boxes predicted by the box head rather than proposal boxes.
_C.MODEL.ROI_BOX_HEAD.TRAIN_ON_PRED_BOXES = False
# ---------------------------------------------------------------------------- #
# Cascaded Box Head
# ---------------------------------------------------------------------------- #
_C.MODEL.ROI_BOX_CASCADE_HEAD = CN()
# The number of cascade stages is implicitly defined by the length of the following two configs.
_C.MODEL.ROI_BOX_CASCADE_HEAD.BBOX_REG_WEIGHTS = (
(10.0, 10.0, 5.0, 5.0),
(20.0, 20.0, 10.0, 10.0),
(30.0, 30.0, 15.0, 15.0),
)
_C.MODEL.ROI_BOX_CASCADE_HEAD.IOUS = (0.5, 0.6, 0.7)
# ---------------------------------------------------------------------------- #
# Mask Head
# ---------------------------------------------------------------------------- #
_C.MODEL.ROI_MASK_HEAD = CN()
_C.MODEL.ROI_MASK_HEAD.NAME = "MaskRCNNConvUpsampleHead"
_C.MODEL.ROI_MASK_HEAD.POOLER_RESOLUTION = 14
_C.MODEL.ROI_MASK_HEAD.POOLER_SAMPLING_RATIO = 0
_C.MODEL.ROI_MASK_HEAD.NUM_CONV = 0 # The number of convs in the mask head
_C.MODEL.ROI_MASK_HEAD.CONV_DIM = 256
# Normalization method for the convolution layers.
# Options: "" (no norm), "GN", "SyncBN".
_C.MODEL.ROI_MASK_HEAD.NORM = ""
# Whether to use class agnostic for mask prediction
_C.MODEL.ROI_MASK_HEAD.CLS_AGNOSTIC_MASK = False
# Type of pooling operation applied to the incoming feature map for each RoI
_C.MODEL.ROI_MASK_HEAD.POOLER_TYPE = "ROIAlignV2"
# ---------------------------------------------------------------------------- #
# Keypoint Head
# ---------------------------------------------------------------------------- #
_C.MODEL.ROI_KEYPOINT_HEAD = CN()
_C.MODEL.ROI_KEYPOINT_HEAD.NAME = "KRCNNConvDeconvUpsampleHead"
_C.MODEL.ROI_KEYPOINT_HEAD.POOLER_RESOLUTION = 14
_C.MODEL.ROI_KEYPOINT_HEAD.POOLER_SAMPLING_RATIO = 0
_C.MODEL.ROI_KEYPOINT_HEAD.CONV_DIMS = tuple(512 for _ in range(8))
_C.MODEL.ROI_KEYPOINT_HEAD.NUM_KEYPOINTS = 17 # 17 is the number of keypoints in COCO.
# Images with too few (or no) keypoints are excluded from training.
_C.MODEL.ROI_KEYPOINT_HEAD.MIN_KEYPOINTS_PER_IMAGE = 1
# Normalize by the total number of visible keypoints in the minibatch if True.
# Otherwise, normalize by the total number of keypoints that could ever exist
# in the minibatch.
# The keypoint softmax loss is only calculated on visible keypoints.
# Since the number of visible keypoints can vary significantly between
# minibatches, this has the effect of up-weighting the importance of
# minibatches with few visible keypoints. (Imagine the extreme case of
# only one visible keypoint versus N: in the case of N, each one
# contributes 1/N to the gradient compared to the single keypoint
# determining the gradient direction). Instead, we can normalize the
# loss by the total number of keypoints, if it were the case that all
# keypoints were visible in a full minibatch. (Returning to the example,
# this means that the one visible keypoint contributes as much as each
# of the N keypoints.)
_C.MODEL.ROI_KEYPOINT_HEAD.NORMALIZE_LOSS_BY_VISIBLE_KEYPOINTS = True
# Multi-task loss weight to use for keypoints
# Recommended values:
# - use 1.0 if NORMALIZE_LOSS_BY_VISIBLE_KEYPOINTS is True
# - use 4.0 if NORMALIZE_LOSS_BY_VISIBLE_KEYPOINTS is False
_C.MODEL.ROI_KEYPOINT_HEAD.LOSS_WEIGHT = 1.0
# Type of pooling operation applied to the incoming feature map for each RoI
_C.MODEL.ROI_KEYPOINT_HEAD.POOLER_TYPE = "ROIAlignV2"
# ---------------------------------------------------------------------------- #
# Semantic Segmentation Head
# ---------------------------------------------------------------------------- #
_C.MODEL.SEM_SEG_HEAD = CN()
_C.MODEL.SEM_SEG_HEAD.NAME = "SemSegFPNHead"
_C.MODEL.SEM_SEG_HEAD.IN_FEATURES = ["p2", "p3", "p4", "p5"]
# Label in the semantic segmentation ground truth that is ignored, i.e., no loss is calculated for
# the correposnding pixel.
_C.MODEL.SEM_SEG_HEAD.IGNORE_VALUE = 255
# Number of classes in the semantic segmentation head
_C.MODEL.SEM_SEG_HEAD.NUM_CLASSES = 54
# Number of channels in the 3x3 convs inside semantic-FPN heads.
_C.MODEL.SEM_SEG_HEAD.CONVS_DIM = 128
# Outputs from semantic-FPN heads are up-scaled to the COMMON_STRIDE stride.
_C.MODEL.SEM_SEG_HEAD.COMMON_STRIDE = 4
# Normalization method for the convolution layers. Options: "" (no norm), "GN".
_C.MODEL.SEM_SEG_HEAD.NORM = "GN"
_C.MODEL.SEM_SEG_HEAD.LOSS_WEIGHT = 1.0
_C.MODEL.PANOPTIC_FPN = CN()
# Scaling of all losses from instance detection / segmentation head.
_C.MODEL.PANOPTIC_FPN.INSTANCE_LOSS_WEIGHT = 1.0
# options when combining instance & semantic segmentation outputs
_C.MODEL.PANOPTIC_FPN.COMBINE = CN({"ENABLED": True})
_C.MODEL.PANOPTIC_FPN.COMBINE.OVERLAP_THRESH = 0.5
_C.MODEL.PANOPTIC_FPN.COMBINE.STUFF_AREA_LIMIT = 4096
_C.MODEL.PANOPTIC_FPN.COMBINE.INSTANCES_CONFIDENCE_THRESH = 0.5
# ---------------------------------------------------------------------------- #
# RetinaNet Head
# ---------------------------------------------------------------------------- #
_C.MODEL.RETINANET = CN()
# This is the number of foreground classes.
_C.MODEL.RETINANET.NUM_CLASSES = 80
_C.MODEL.RETINANET.IN_FEATURES = ["p3", "p4", "p5", "p6", "p7"]
# Convolutions to use in the cls and bbox tower
# NOTE: this doesn't include the last conv for logits
_C.MODEL.RETINANET.NUM_CONVS = 4
# IoU overlap ratio [bg, fg] for labeling anchors.
# Anchors with < bg are labeled negative (0)
# Anchors with >= bg and < fg are ignored (-1)
# Anchors with >= fg are labeled positive (1)
_C.MODEL.RETINANET.IOU_THRESHOLDS = [0.4, 0.5]
_C.MODEL.RETINANET.IOU_LABELS = [0, -1, 1]
# Prior prob for rare case (i.e. foreground) at the beginning of training.
# This is used to set the bias for the logits layer of the classifier subnet.
# This improves training stability in the case of heavy class imbalance.
_C.MODEL.RETINANET.PRIOR_PROB = 0.01
# Inference cls score threshold, only anchors with score > INFERENCE_TH are
# considered for inference (to improve speed)
_C.MODEL.RETINANET.SCORE_THRESH_TEST = 0.05
_C.MODEL.RETINANET.TOPK_CANDIDATES_TEST = 1000
_C.MODEL.RETINANET.NMS_THRESH_TEST = 0.5
# Weights on (dx, dy, dw, dh) for normalizing Retinanet anchor regression targets
_C.MODEL.RETINANET.BBOX_REG_WEIGHTS = (1.0, 1.0, 1.0, 1.0)
# Loss parameters
_C.MODEL.RETINANET.FOCAL_LOSS_GAMMA = 2.0
_C.MODEL.RETINANET.FOCAL_LOSS_ALPHA = 0.25
_C.MODEL.RETINANET.SMOOTH_L1_LOSS_BETA = 0.1
# ---------------------------------------------------------------------------- #
# ResNe[X]t options (ResNets = {ResNet, ResNeXt}
# Note that parts of a resnet may be used for both the backbone and the head
# These options apply to both
# ---------------------------------------------------------------------------- #
_C.MODEL.RESNETS = CN()
_C.MODEL.RESNETS.DEPTH = 50
_C.MODEL.RESNETS.OUT_FEATURES = ["res4"] # res4 for C4 backbone, res2..5 for FPN backbone
# Number of groups to use; 1 ==> ResNet; > 1 ==> ResNeXt
_C.MODEL.RESNETS.NUM_GROUPS = 1
# Options: FrozenBN, GN, "SyncBN", "BN"
_C.MODEL.RESNETS.NORM = "FrozenBN"
# Baseline width of each group.
# Scaling this parameters will scale the width of all bottleneck layers.
_C.MODEL.RESNETS.WIDTH_PER_GROUP = 64
# Place the stride 2 conv on the 1x1 filter
# Use True only for the original MSRA ResNet; use False for C2 and Torch models
_C.MODEL.RESNETS.STRIDE_IN_1X1 = True
# Apply dilation in stage "res5"
_C.MODEL.RESNETS.RES5_DILATION = 1
# Output width of res2. Scaling this parameters will scale the width of all 1x1 convs in ResNet
_C.MODEL.RESNETS.RES2_OUT_CHANNELS = 256
_C.MODEL.RESNETS.STEM_OUT_CHANNELS = 64
# Apply Deformable Convolution in stages
# Specify if apply deform_conv on Res2, Res3, Res4, Res5
_C.MODEL.RESNETS.DEFORM_ON_PER_STAGE = [False, False, False, False]
# Use True to use modulated deform_conv (DeformableV2, https://arxiv.org/abs/1811.11168);
# Use False for DeformableV1.
_C.MODEL.RESNETS.DEFORM_MODULATED = False
# Number of groups in deformable conv.
_C.MODEL.RESNETS.DEFORM_NUM_GROUPS = 1
# ---------------------------------------------------------------------------- #
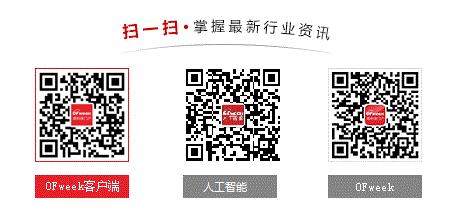
最新活动更多
-
2月25日火热报名中>> Ansys Motion薄膜卷曲卷对卷工艺仿真解决方案
-
2月28日火热报名中>> 【免费试用】东集技术年终福利——免费试用活动
-
即日-3.21立即报名 >> 【深圳 IEAE】2025 消费新场景创新与实践论坛
-
3月27日立即报名>> 【工程师系列】汽车电子技术在线大会
-
4日10日立即报名>> OFweek 2025(第十四届)中国机器人产业大会
-
7.30-8.1火热报名中>> 全数会2025(第六届)机器人及智能工厂展
推荐专题
-
10 百度AI,恨铁不成钢
发表评论
请输入评论内容...
请输入评论/评论长度6~500个字
暂无评论
暂无评论